In today’s rapidly evolving landscape, technology is the catalyst for transforming field operations—from chaotic scheduling and routing to intelligent, optimized dispatching. In our series on dispatch software evolution, we explored the journey from cumbersome manual processes to fully automated systems that drive efficiency and performance. This installment focuses on how integrating AI in dispatch software can further empower organizations to achieve breakthrough improvements in operational efficiency, customer satisfaction, and bottom-line impact.
This blog is geared towards a broad spectrum of decision-makers, including CEOs, operations managers, field service managers, and professionals in sectors such as HVAC, home appliances, medical equipment manufacturing, solar panel installation, and more. Even junior team members and industry peers from Field Service Management (FSM) product companies will find actionable insights that resonate with their daily challenges. Read on to learn how to harness artificial intelligence and machine learning technologies to modernize your dispatch operations.
1. Setting the Stage: Why AI and Machine Learning?
The current landscape of field service operations demands agility, precision, and intelligent response to unpredictable conditions. Traditional dispatch models have long struggled under the weight of manual scheduling, routing complexities, and reactive decision-making. With AI in dispatch software, organizations are empowered to leverage advanced computing to predict, analyze, and optimize every aspect of field operations.
Key Considerations
- Data-Driven Decision Making: The wealth of information generated by field operations can be transformed into actionable insights with machine learning algorithms. By analyzing historical data alongside real-time input, these systems guide smarter decision-making.
- Enhanced Efficiency: Integrating predictive scheduling and machine learning routing allows companies to minimize travel times, reduce delays, and maximize technician productivity.
- Competitive Advantage: In industries where customer responsiveness and service quality are critical, AI-driven field service solutions create a distinct edge over the competition.
As we move through this article, you will discover step-by-step guidance on implementing these technologies to overcome common challenges and pave the way for a more streamlined and effective dispatch system.
2. Understanding the Components: AI and Machine Learning in Dispatch Software
What Are AI and Machine Learning in This Context?
At their core, artificial intelligence and machine learning mimic human decision-making processes by analyzing patterns in data. In dispatch software, they enable:
- Predictive Scheduling: Leveraging historical records to forecast service demands, thereby anticipating workload fluctuations before they occur. This results in not just reactive, but proactive scheduling that optimizes manpower allocation.
- Machine Learning Routing: Using algorithms that learn from past routing data to craft more efficient travel paths and routing decisions, adapting over time to traffic, weather, and service-specific nuances.
- Intelligent Dispatch Algorithms: Advanced algorithms dynamically assign tasks based on a multitude of factors, such as technician skills, location, availability, and real-time updates.
- Smart Dispatch Analytics: Real-time data visualization and analysis allow managers to monitor key performance metrics and adjust strategies on the fly.
How Do They Work Together?
The integration of these components is not just about automating existing processes—it’s about reimagining how field operations operate. AI systems can:
- Analyze multi-source data (including weather updates, traffic patterns, and technician performance) to optimize dispatch decisions.
- Self-learn and improve over time, meaning that the more the system operates, the better it becomes at predicting outcomes and recommending actions.
- Deliver actionable insights that help decision-makers preempt challenges and seize opportunities to enhance service delivery.
Understanding these elements is the first step towards a robust, AI-enhanced dispatch software environment. In the sections that follow, we dive deeper into actionable strategies for implementing these advanced tools.
3. How to Implement Predictive Scheduling in Your Dispatch Operations
One of the most transformative features of AI in dispatch software is the ability to anticipate service demands and schedule resources accordingly. Here’s how to get started:
Step-by-Step Guide for Predictive Scheduling
- Data Collection and Preparation:
- Begin by consolidating historical service data from all your operations. This includes service call logs, customer feedback, technician performance records, and environmental factors.
- Ensure data accuracy and completeness. Data cleansing and normalization are critical to build a reliable prediction model.
- Choosing the Right Tools:
- Evaluate AI platforms that provide robust analytics capabilities and integrate seamlessly with your existing dispatch system.
- Look for tools that offer customizable dashboards so you can monitor key metrics and performance indicators.
- Building Predictive Models:
- Use statistical and machine learning methods to identify patterns in service requests. Algorithms such as regression analysis or time-series forecasting can be particularly effective.
- Continuously train your model with new data to enhance its accuracy over time.
- Integration with Dispatch Software:
- Seamlessly embed predictive scheduling features into your current dispatch system. This could be a modular integration or a full-scale platform migration.
- Ensure that the system provides real-time alerts and notifications to allow for dynamic adjustments during peak times or unexpected surges.
- Testing and Feedback:
- Pilot the system with a subset of operations. Collect performance metrics such as response times, service levels, and technician workload.
- Solicit feedback from the field team and adjust the model as needed for improved precision.
By following these steps, operations managers can transform reactive scheduling practices into proactive, data-driven planning, ensuring optimal resource utilization and enhanced customer satisfaction.
4. Mastering Machine Learning Routing for Enhanced Efficiency
Machine learning routing is another critical aspect that leverages real-time data to enhance dispatch effectiveness. Here’s how you can fine-tune your routing strategy:
Practical Steps for Advanced Routing
- Leverage Historical Routing Data:
- Analyze past routes to understand patterns such as high-traffic periods and common bottlenecks.
- Identify variables that impact routing decisions, such as geographic factors and service-specific constraints.
- Integrate Real-Time Updates:
- Utilize real-time data streams for traffic, weather conditions, and road closures.
- Implement routing algorithms that adapt on the fly, recalculating paths and suggesting alternate routes if conditions deteriorate.
- Emphasize Learning and Adaptation:
- Configure the system to learn from each trip. The more data it processes, the better it can predict optimal routes under varying conditions.
- Monitor the success of algorithm adjustments and refine the parameters for improved accuracy.
- Combine Routing with Scheduling Efforts:
- Coordinate routing decisions with your predictive scheduling models so that technicians are not only scheduled at the right time but also directed via the most efficient paths.
- Ensure your dispatch software can reconcile these two processes to provide holistic recommendations.
- Evaluate Performance:
- Track key performance indicators such as travel time reduction, cost savings on fuel, and improvements in service times.
- Use these insights to further optimize the machine learning routing protocols.
By harnessing machine learning routing, companies achieve quicker turnaround times and a smoother allocation of resources, ultimately leading to improved operational efficiency and a more agile field service environment.
5. Leveraging AI-Driven Field Service for Business Impact
The transition towards AI-driven field service is paving the way for smarter, more agile operations. This transformation extends beyond improved scheduling and routing—it touches every facet of dispatch operations.
How AI-Driven Field Service Transforms Your Business
- Resource Optimization:
AI-powered systems adjust not only schedules and routes but also recommend the best allocation of resources based on real-time changes and evolving priorities. - Enhanced Customer Experience:
Faster response times, proactive maintenance alerts, and more accurate service windows contribute to higher customer satisfaction levels. - Cost Reduction:
By optimizing routes and schedules, companies reduce fuel consumption, minimize overtime, and lower overall operational costs. - Scalability:
Intelligent dispatch software can easily scale to accommodate seasonal peaks or rapid business expansion, ensuring consistency in service quality regardless of volume.
Real-World Benefits
Consider the case of an HVAC service provider faced with seasonal surges. With AI in dispatch software, they could predict peak periods based on historical data, adjusting technician schedules accordingly. Simultaneously, intelligent dispatch algorithms would determine the optimal routing, considering live traffic and weather conditions. The result? A noticeable reduction in downtime, enhanced service reliability, and improved customer trust—all vital metrics that impact the bottom line.
Such real-world scenarios illustrate how AI-driven field service not only streamlines operations but also drives significant business outcomes, from cost savings to enhanced service quality.
6. Integrating Intelligent Dispatch Algorithms and Smart Dispatch Analytics
Intelligent Dispatch Algorithms: A Closer Look
At the heart of next-generation dispatch systems are intelligent dispatch algorithms. These algorithms go beyond simple rule-based logic by incorporating multiple parameters simultaneously:
- Technician Proficiency: Assigning tasks based on skillset and historical performance.
- Geolocation Data: Optimizing travel routes using live mapping data.
- Workload Balancing: Ensuring equitable distribution of tasks to prevent overload and burnout.
- Customer Prioritization: Recognizing high-priority service requests and dynamically adjusting schedules accordingly.
The benefits of implementing these algorithms include more precise service delivery, reduced idle time, and better overall field management. Decision-makers can rely on these systems to make complex dispatch decisions with minimal manual intervention.
The Power of Smart Dispatch Analytics
Complementary to the algorithms, smart dispatch analytics provide managers with deep insights into operational performance. Here’s how to make the most of it:
- Dashboards and Visualizations:
- Use analytics dashboards that consolidate key metrics—ranging from response times to technician productivity—into a single view.
- Interactive visualizations allow for fast identification of performance trends and potential issues.
- Predictive and Prescriptive Insights:
- Advanced analytics move beyond descriptive reporting. They forecast future patterns and recommend actionable steps.
- With insights powered by AI, managers can foresee issues before they escalate and implement solutions swiftly.
- Continuous Improvement:
- Regularly review analytics reports to pinpoint areas for improvement.
- Use the insights to feed back into your predictive scheduling and machine learning routing systems, ensuring they evolve continuously.
Integrating intelligent dispatch algorithms with smart dispatch analytics creates a feedback loop, where operational performance is constantly measured, refined, and improved. This holistic approach not only transforms dispatch processes but also positions organizations to react dynamically to an ever-changing field environment.
7. How to Successfully Integrate AI and Machine Learning Into Your Dispatch Software
Transitioning to an AI-enhanced dispatch operation requires a well-planned, step-by-step approach. Here’s a structured guide to help your organization make the move:
Step 1: Conduct a Comprehensive Audit
- Assess Current Capabilities:
Start by evaluating your current dispatch processes and software capabilities. Identify areas that are heavily reliant on manual inputs and those that could benefit most from automation. - Data Inventory:
Catalog all available operational data, including historical service records, technician performance, and external data sources (e.g., traffic, weather).
Step 2: Define Clear Objectives
- Business Outcomes:
Outline what you want to achieve: reduced downtime, improved customer satisfaction, cost savings, or enhanced technician productivity. - Performance Metrics:
Establish KPIs that will help measure the success of AI implementations. These might include faster response times, improved route efficiency, and higher service completion rates.
Step 3: Choose Your Technology Partners
- Vendor Evaluation:
Look for technology providers who specialize in AI in dispatch software and who can demonstrate successful case studies in industries similar to yours. - Integration Capability:
Ensure that the chosen solution integrates smoothly with your existing systems, such as CRM, ERP, and other field service management tools.
Step 4: Pilot Implementation and Training
- Start Small:
Test the AI functionalities on a smaller scale or in a pilot region. This minimizes risk and provides a controlled environment for adjustments. - Staff Training:
Equip managers and technicians with necessary training. A solid understanding of new systems fosters smoother adoption and helps in harvesting accurate feedback.
Step 5: Rollout and Monitor
- Iterative Implementation:
Gradually expand the system’s usage across your operations. Monitor performance closely and tweak the system based on real-world results. - Feedback Loop:
Create channels for continuous feedback from field teams and integrate their insights to refine the predictive models, routing algorithms, and overall system performance.
Step 6: Analyze, Adjust, and Scale
- Data-Driven Decisions:
Continuously leverage smart dispatch analytics to assess performance. Adjust the algorithms and strategies based on emerging trends and anomalies. - Scalability Planning:
As you scale up, plan for system expansions that can handle increased data loads and more complex dispatch scenarios without compromising on efficiency.
Following this methodical integration process ensures that your transition to an AI-enhanced dispatch environment is smooth, sustainable, and aligned with your strategic objectives.
8. Industry-Specific Considerations and Use Cases
While the technological aspects of AI and machine learning are universally applicable, its real-world impact often depends on industry-specific nuances. Here are some examples to illustrate the diverse benefits:
HVAC and Home Appliances
- Seasonal Demand and Maintenance:
In industries like HVAC, demand for service can be highly seasonal. Predictive scheduling helps anticipate peak times, ensuring enough technicians are available during extreme weather periods. - Efficient Routing:
Machine learning routing minimizes the time technicians spend traveling between service calls, significantly reducing costs while ensuring prompt service.
Medical Equipment Manufacturers
- Critical Service Requirements:
For companies servicing medical equipment, timely maintenance is a matter of life and death. AI-driven models prioritize urgent calls and optimize routes to ensure that the right technician reaches the site immediately. - Compliance and Reporting:
Smart dispatch analytics provide detailed logs and performance reports, which are crucial for adhering to regulatory requirements and ensuring the highest service standards.
Solar Panel Installation Firms
- Project Management:
These firms often deal with large-scale installations that require synchronized coordination of multiple teams. AI can help in predicting project timelines and managing resource allocation. - Cost Efficiency:
Optimized routing and scheduling translate directly into reduced installation times and lower operational costs, boosting overall profitability.
Across these industries, the common thread is the ability of AI in dispatch software to transform operational challenges into measurable business improvements, ensuring that service quality and efficiency remain at the forefront.
9. Future Trends and Next Steps
As technology continues to evolve, so too will the capabilities of dispatch software. Looking forward, several emerging trends are likely to shape the future of AI-powered dispatch operations:
Anticipated Developments
- Hyper-Personalization:
Future systems may provide even more tailored service experiences by integrating customer preferences and feedback into real-time decision-making. - Autonomous Field Operations:
The convergence of AI with Internet of Things (IoT) devices and robotics could lead to semi-autonomous service operations, reducing manual intervention even further. - Enhanced Security Measures:
With increasing reliance on data-driven systems, cybersecurity will be paramount. Expect advanced AI-based security protocols to be integrated into dispatch software. - Integration with Broader Business Intelligence:
As businesses move toward fully integrated ecosystems, dispatch software will likely become a central hub that interacts seamlessly with inventory management, supply chain systems, and overall business analytics.
Preparing for the Future
To stay ahead, organizations should invest in ongoing training and development, foster a culture of innovation, and continuously review their AI strategies. Adapting to new technologies early on can unlock further efficiencies and position companies as industry leaders in field service management.
Embrace the Future of Dispatch
Adopting AI in dispatch software is not merely about embracing cutting-edge technology—it’s about rethinking and redesigning your entire field service model. By integrating predictive scheduling, machine learning routing, AI-driven field service, intelligent dispatch algorithms, and smart dispatch analytics, organizations have the power to drive significant improvements in operational efficiency, customer satisfaction, and overall profitability.
Whether you are a CEO looking to transform business strategy, an operations manager dedicated to optimizing workflows, or a field service leader tasked with daily challenges, the roadmap outlined here provides clear, actionable steps to harness the full potential of AI. As you integrate these technologies, remember to adapt continuously, leverage real-time insights, and create a feedback loop that fuels further innovation.
With the right approach, AI and machine learning will not only enhance your dispatch software but also fundamentally redefine how your business operates in an increasingly complex and dynamic market. Embrace these innovations today to build a smarter, more efficient tomorrow.
Book a demo Free today and see how Fieldax can revolutionize your dispatch process.
Author Bio
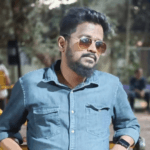
Palani Kumar
Palani Kumar is a seasoned digital marketing professional with over 13 years of experience in crafting impactful strategies for B2B brands.
As the marketing lead at FieldAx, he focuses on bridging the gap between technology and business growth, simplifying complex field service management concepts into valuable insights.
Passionate about delivering informative and practical content, Palani writes about industry trends, best practices, and innovations that help businesses optimize their field operations.
When not working on marketing strategies, he enjoys exploring history, engaging in thought-provoking discussions, and appreciating the balance between technology and tradition.