Modern HVAC operations face mounting pressures to minimize unplanned downtime, control soaring service costs, and elevate overall system reliability. In this landscape, Predictive Analysis offers a paradigm shift: by harnessing real-time sensor feeds and historical maintenance records, organizations can anticipate equipment issues before they escalate into costly emergencies.
At its core, predictive analytics blends machine-learning models with live performance data—transforming raw signals from compressors, chillers, and air handlers into actionable insights. Rather than following rigid, calendar-based schedules, field teams gain the ability to intervene precisely when a component’s health indicates looming degradation, preventing failures and extending asset lifecycles.
This evolution reshapes the very fabric of field service management. Work orders spring to life from automated alerts, technicians arrive fully equipped with the right parts, and maintenance plans adapt dynamically as conditions change. Underpinning this shift is robust data analytics: dashboards that visualize asset health, algorithms that forecast workload peaks, and continuous feedback loops that refine prediction accuracy over time.
In the sections that follow, we’ll explore how embedding these capabilities into your service operations drives measurable ROI, boosts first-time fix rates, and lays the groundwork for truly proactive, high-performance HVAC maintenance.
The Evolution of HVAC Field Service Management
In the early days, HVAC technicians operated almost entirely in reactive mode—responding to emergency breakdowns and customer complaints as they arose. This ad hoc approach often led to prolonged equipment downtime, rushed repairs, and ballooning costs. Over time, organizations embraced preventive maintenance, scheduling routine checks and part replacements at fixed intervals. While this reduced catastrophic failures, it still relied on calendar-based cycles rather than actual asset condition, resulting in unnecessary service visits and underutilized maintenance windows.
As customer expectations for uptime and comfort levels have intensified, and as HVAC systems grow more complex with integrated controls, variable-speed drives, and IoT-enabled components, companies have turned to digital solutions to stay ahead. The shift toward a software for field service management has enabled centralized dispatching, mobile work-order access, and real-time job tracking. These platforms bridge the gap between the office and the field, equipping technicians with immediate access to equipment history, parts catalogs, and service protocols, all from their smartphones or tablets.
At the heart of this transformation lies robust maintenance management software, which aggregates data from connected devices, building management systems, and service logs into one unified dashboard. A well-integrated customer service management system further enhances visibility by tracking customer preferences, SLA requirements, and job feedback. Together, these technologies enable a proactive service model—where alerts trigger work orders only when performance metrics breach predefined thresholds, parts are ordered just in time, and resources are dispatched optimally. By evolving from reactive fixes to preventive routines and now to condition-based interventions, HVAC organizations are driving higher efficiency, lower operating costs, and elevated customer satisfaction.
Predictive Analytics: Smarter Decisions from Real-Time Data
Predictive Analysis represents a leap beyond calendar-based upkeep. While preventive maintenance relies on fixed schedules, predictive analytics uses live equipment data—temperature, vibration, and pressure readings—to forecast issues before they emerge. By continuously assessing machine health, organizations replace guesswork with precision, intervening only when an asset’s condition signals degradation.
Key technologies power this shift. IoT sensors feed raw signals into centralized platforms designed for performance monitoring, tracking metrics such as compressor RPM and refrigerant flow. These streams are then processed by advanced analytics tools—from trend-analysis dashboards to anomaly-detection algorithms—that flag outliers and patterns indicative of wear. At the core lie machine-learning models that drive predictive data analytics, learning from historical failures to predict future breakdowns and automatically generating work orders when thresholds are breached.
Underpinning all this is rigorous data management. Quality forecasting demands clean, consistent datasets: standardized naming conventions for equipment, complete maintenance logs, and synchronized time stamps across sensors. Without strong data governance—defining ownership, validation rules, and secure storage—models misfire, and false alerts erode trust.
According to McKinsey & Company, predictive maintenance programs typically reduce machine downtime by 30–50% and extend equipment life by 20–40% McKinsey & Company. In the chapters ahead, we’ll explore how to assemble these technologies into an integrated FSM strategy, turning raw data into actionable insights and sustainable competitive advantage.
Why It Matters: Real Results from Predictive Maintenance
Reduced Downtime & Costs. By leveraging real-time performance monitoring and machine-learning alerts, organizations can detect early signs of component wear—such as abnormal vibration or temperature spikes—and schedule interventions before failures occur. McKinsey & Company reports that predictive maintenance can reduce machine downtime by 30–50% and extend equipment life by 20–40% McKinsey & Company. For HVAC, this translates into fewer emergency call-outs, lower overtime labor costs, and avoidance of expensive replacement parts.
Smarter Dispatch. Integrating smart routing algorithms into your FSM platform ensures technicians arrive on time with the right tools. Route optimization minimizes travel distances and idle time, while an embedded workflow management software module automates job assignment based on skill set, part availability, and geographic proximity. The result is faster response times, higher first-time fix rates, and reduced fuel expenses.
Optimized Inventory. Predictive analytics can forecast part failures days or weeks in advance, feeding your inventory management and IT inventory management systems with precise reorder points. Rather than stocking excess spares or risking stock-outs, you maintain an optimal parts level—saving carrying costs and ensuring technicians never leave a job site in search of components.
Elevated Customer Experience. Today’s clients expect transparent service and rapid resolution. An integrated customer support solutions platform ties service history, SLAs, and real-time equipment health data into a unified interface. Automated notifications keep customers informed of upcoming maintenance windows, while service teams can access past work orders and customer preferences on their mobile devices—boosting satisfaction and loyalty.
Together, these capabilities redefine HVAC field service from a reactive cost center into a proactive profit driver. By combining sensor-driven insights with intelligent dispatch, just-in-time inventory, and seamless customer communications, organizations unlock efficiency gains, drive down operating expenses, and deliver premium service quality in every engagement.
Tech Stack Strategy: What to Look For in FSM Software
Selecting the ideal software solution is critical for realizing the full potential of predictive analytics. At the entry level, hvac field service management software provides mobile work-order access, basic scheduling, and equipment histories. It’s tailored to HVAC-specific workflows but may lack advanced analytics modules. A step up, hvac service management software often includes deeper maintenance planning features and integration with building management systems, enabling richer data capture and streamlined preventive maintenance.
For organizations seeking end-to-end visibility, all-in-one software for field service management suites combine CRM, ERP, and IoT data feeds into a unified platform. These comprehensive solutions can ingest real-time sensor data, automate parts replenishment, and generate service contracts—all from a single dashboard. The trade-off is higher implementation complexity and upfront cost, so it’s vital to assess your team’s technical capacity and change management readiness.
Integration requirements should guide your vendor evaluation. Ensure seamless connectivity with your existing CRM to maintain customer profiles and SLA commitments. ERP integration is essential for financial reconciliation and parts costing, while open APIs for IoT data feeds allow live performance monitoring and device health tracking. Look for platforms that support standard protocols (e.g., MQTT, OPC UA) to avoid vendor lock-in.
When it comes to predictive maintenance tools, key evaluation criteria include real-time alerting capabilities, customizable dashboards, and ML-driven forecasting accuracy. Prefer solutions offering built-in anomaly detection models that can be retrained on your historical data, as well as visual performance trends over customizable time windows. A user-friendly interface for setting threshold alerts and receiving mobile notifications ensures technicians and managers stay aligned. By carefully weighing platform scope, integration fit, and predictive feature sets, you’ll equip your organization with the right stack to drive Continuous Improvement and operational excellence.
From Data to Deployment: Your 4-Step Predictive FSM Blueprint
- Data Audit & Cleanup
Begin by inventorying all your data sources—IoT sensor feeds, CMMS logs, and historical service records—and standardize naming conventions, time stamps, and equipment identifiers. Establish validation rules to catch anomalies (e.g., out-of-range temperature readings) and fill in missing values where possible. This foundational step ensures your data analytics engines receive consistent, high-quality inputs, preventing model drift and false alarms down the road. - Pilot & Scale
Select a manageable subset of high-impact assets—such as critical chillers or rooftop units—and deploy your predictive models in a controlled environment. Monitor model accuracy and alert relevance over a 4–6-week period, comparing predicted failures against actual maintenance events. Use these insights to recalibrate thresholds and retrain algorithms. Once the pilot demonstrates a clear ROI (e.g., reduced emergency repairs or extended component lifespans), gradually roll out to additional sites and equipment types. - Train Field Technicians
Equip each field technician with mobile access to real-time alerts, equipment histories, and parts requirements. Conduct hands-on workshops demonstrating how to interpret prediction scores, log findings, and initiate corrective actions through your chosen platform. Encourage feedback loops—technicians should report false positives or missing context so your team can refine model parameters and ensure high adoption rates. - Continuous Improvement
Predictive models are not “set and forget.” Establish a quarterly review process to assess model performance, incorporate new failure modes, and integrate fresh data streams (e.g., weather or occupancy patterns). Refine your maintenance management software workflows by automating repetitive tasks—like parts ordering—and expanding rule sets to cover emerging insights.
Throughout all phases, strong service management governance is critical. Define clear ownership of data assets, model stewardship responsibilities, and escalation paths for critical alerts. By embedding accountability and iterative checks into your roadmap, you’ll ensure that predictive analytics remains a trusted, evolving cornerstone of your HVAC field service operations.
What’s Next: Tomorrow’s Tools Shaping HVAC Service
AI-Driven Recommendations
As machine-learning models mature, HVAC service platforms will deliver contextual, AI-driven suggestions for both emergent and scheduled jobs. Advanced anomaly-detection algorithms not only flag off-nominal readings but also recommend corrective actions—whether it’s adjusting refrigerant charge, cleaning coils, or replacing bearings. These next-gen models learn from each service event, refining their accuracy and expanding their knowledge base automatically. Over time, they’ll anticipate not just component failures but the optimal maintenance window, balancing technician availability, parts inventory, and customer preferences.
Edge Analytics
Shifting computation to the device level enables true real-time insights. With on-device predictive data analytics, sensors and controllers analyze streams locally—triggering alerts in milliseconds without waiting for cloud round trips. This reduces latency, minimizes bandwidth usage, and keeps critical decision-making operational even when connectivity is intermittent. Edge-capable gateways can preprocess data, forwarding only aggregated or anomalous events to centralized servers, streamlining your data pipeline and ensuring that both field teams and managers stay informed without delay.
AR-Powered Field Support
Augmented reality is set to transform technician workflows. By wearing AR headsets or using tablet cameras, technicians can overlay live performance metrics directly onto physical equipment—such as temperature gradients on evaporator coils or vibration hotspots on motor mounts. Interactive overlays link to your analytics tools, allowing users to drill down into historical trends or trigger work orders on the spot. This hands-free access to digital documentation and live data accelerates diagnosis, reduces human error, and speeds up first-time fixes.
Looking ahead, we will see even tighter IoT integration, where unified device standards enable plug-and-play connectivity across manufacturers. Analytics tools ecosystems will deepen, offering marketplaces of prebuilt ML models, sensor drivers, and visualization widgets. Together, these innovations promise a future where HVAC field service becomes not just reactive or preventive, but continuously optimized for efficiency, cost-effectiveness, and unmatched customer satisfaction.
Predictive Analysis is reshaping field service management by transforming raw sensor data into proactive maintenance actions that slash downtime, optimize resources, and boost first-time fix rates. By moving beyond reactive and calendar-based routines, organizations gain a measurable competitive edge: fewer emergency repairs, lower operating costs, and stronger customer loyalty.
If you’re ready to unlock these benefits, now is the time to explore leading software for field service management platforms. Request a personalized demo to see real-time performance monitoring, automated work-order generation, and predictive maintenance tools in action. For a low-risk start, consider launching a pilot project on your most critical assets—validate ROI quickly and scale across your operations.
For deeper insight into the forces shaping HVAC service today complete with benchmarks, case studies, and expert forecasts—read our 2025 HVAC Key Market Trends & Strategic Insights report and see exactly how your predictive strategy stacks up.
See how FieldAx can transform your Field Operations.
Try it today! Book Demo
You are one click away from your customized FieldAx Demo!
Author Bio
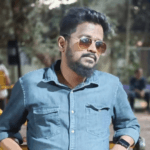
Palani Kumar
Palani Kumar is a seasoned digital marketing professional with over 13 years of experience in crafting impactful strategies for B2B brands.
As the marketing lead at FieldAx, he focuses on bridging the gap between technology and business growth, simplifying complex field service management concepts into valuable insights.
Passionate about delivering informative and practical content, Palani writes about industry trends, best practices, and innovations that help businesses optimize their field operations.
When not working on marketing strategies, he enjoys exploring history, engaging in thought-provoking discussions, and appreciating the balance between technology and tradition.